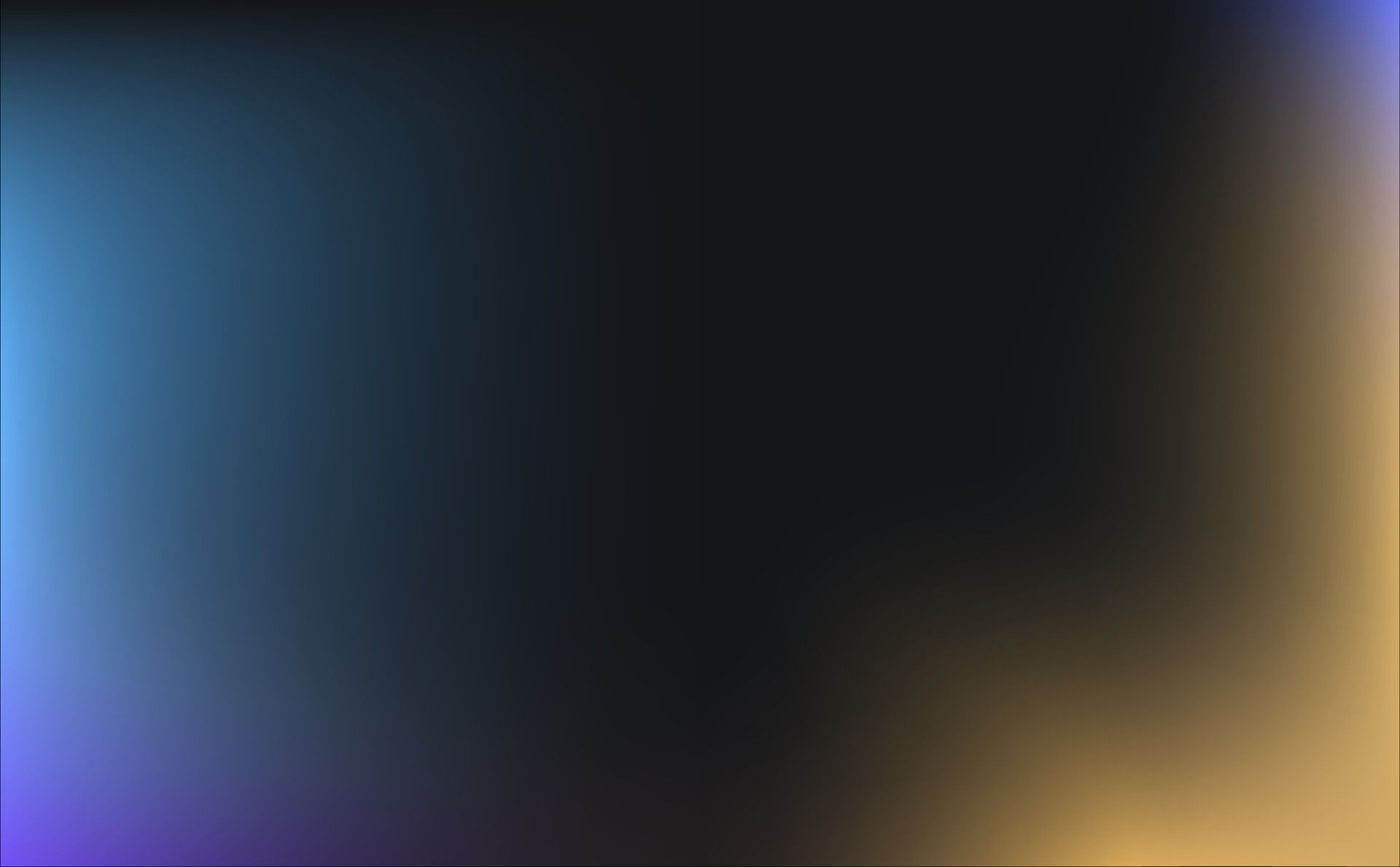
How AI is Reshaping Crypto Trading & Market Making
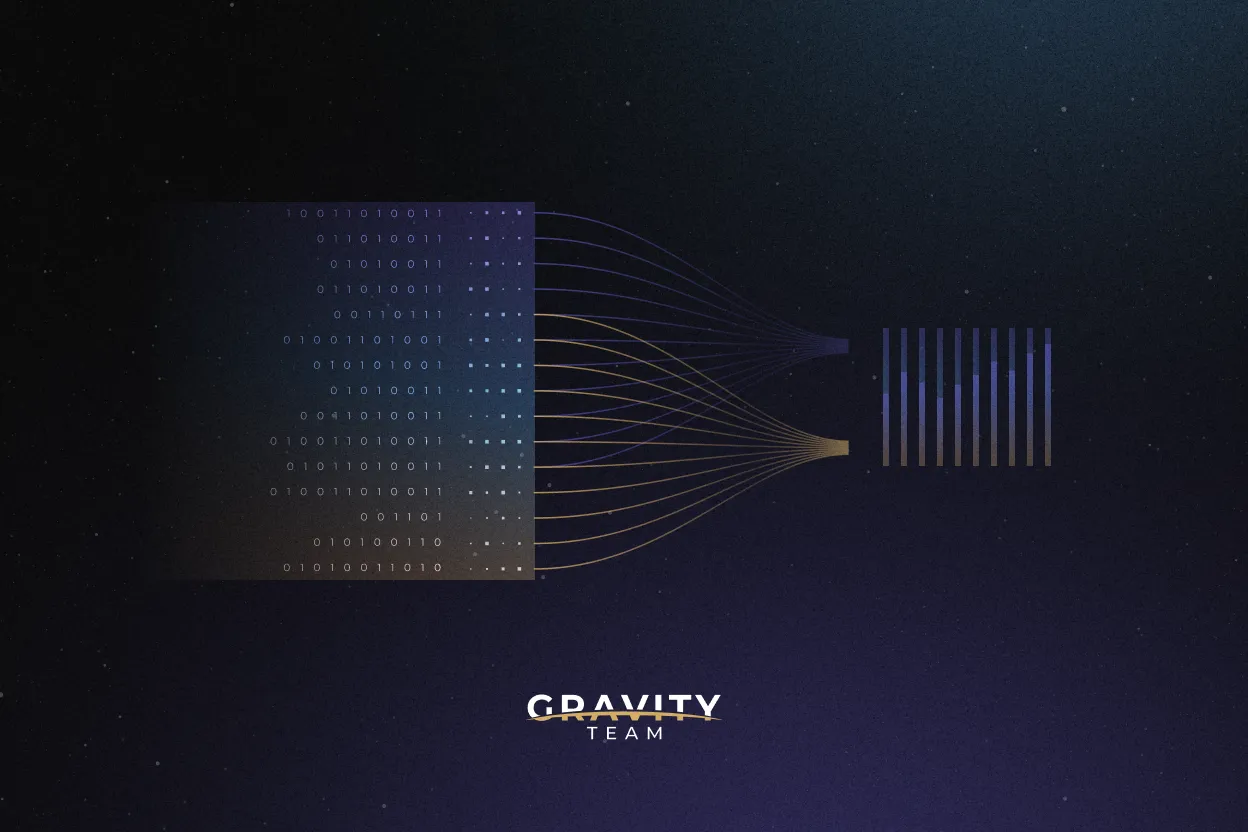
Crypto markets move at breakneck speed, 24/7, across a global network of exchanges. In this arena, algorithmic trading and market making are the engines that keep things running. These algorithmic bots don’t sleep; they execute trades, provide liquidity and narrow spreads to ensure anyone can buy or sell without huge price swings. Market makers play a critical role in stabilising prices and supplying crypto liquidity, much like in traditional markets. The difference? Crypto’s nonstop volatility and fragmented venues demand an even more dynamic, tech-driven approach. This is where Artificial Intelligence (AI) is stepping in, turbocharging algorithmic strategies with machine learning.
AI-driven trading bots process millions of data points in milliseconds to execute crypto trades, far outpacing human reaction times. In 2023, algorithmic crypto trading volume exceeded $94 trillion globally, with bots executing over 70% of all trades. These AI-powered systems crunch vast streams of market data and can fire off orders with as little as 0.01 seconds of latency. Compare that to a human’s 0.1–0.3 second reaction time. In other words, the moment you blink, an AI trading bot could have scanned order books, spotted an arbitrage and executed a profitable trade. Algorithmic market makers, on their side, continuously adjust buy/sell quotes to keep markets liquid. When done right, traders get fast fills and minimal slippage; when liquidity dries up, chaos ensues. The core role of these algorithms is to balance the market: providing depth on both sides and smoothing out the wild swings so that the crypto circus keeps running orderly.
The Core Role of Algorithms in Crypto Markets
At its core, algorithmic trading refers to using pre-programmed rules to execute trades when certain conditions are met automatically. In crypto, algos hunt for opportunities like price inefficiencies, arbitrage gaps between exchanges or short-term momentum plays, all at speeds impossible for any human. Market making, a subset of algorithmic trading, specifically focuses on continuously placing buy and sell orders (quotes) to provide liquidity. A good market maker narrows the bid-ask spread and profits from the spread in return for the service of liquidity provision. In the ultra-volatile crypto environment, this is no simple task. Market makers have to dynamically adjust their quotes in real time to react to big trades and price jumps. They also manage an inventory of coins, trying not to hold too much of one asset that could drop in value suddenly. As Gravity Team and other top firms know, effective market making means juggling risk on one hand and liquidity obligations on the other, a balancing act across dozens of trading pairs and venues.
Crypto market makers aren’t in it for charity; they’re after profit and the health of the market. By stepping in as counter-parties to trades, they prevent situations where no one is on the other side of a buy or sell order. This keeps markets healthy and orderly. In fact, the presence of professional market makers has grown substantially since 2020 as the industry matured. The number of dedicated crypto market-making firms has surged, bringing more professionalism to the space. These firms deploy sophisticated algorithms (and increasingly, AI-driven models) to maintain tight spreads even during turbulent times. The result is that today’s traders on major exchanges often take for granted that they can trade in size at tight spreads, thanks to armies of bots with brains working behind the scenes.
AI: The New Edge in Crypto Trading Strategies
Until recently, most algorithmic strategies followed relatively static logic: if X and Y happen, execute trade Z. Artificial Intelligence is changing that by giving trading bots the ability to learn, adapt and optimize beyond their initial programming. AI comes in many flavours in trading: from deep learning models that forecast prices, to reinforcement learning agents that dynamically adjust strategies through trial-and-error, to large language models that gauge market sentiment from news and tweets. Here’s how AI is elevating crypto trading:
- Deep Learning for Market Prediction: Deep neural networks can digest enormous amounts of historical price data, technical indicators and order book data to identify complex patterns humans might miss. For example, AI algorithms now analyze historical price series, order flow and other metrics to predict future market moves, giving traders a heads-up on trends before they form. Unlike a simple trend-following bot, a deep learning model might discover non-linear relationships, say, between Bitcoin’s volume spikes and altcoin price moves to anticipate price shifts with greater accuracy. Notably, new research in 2025 found that deep learning and NLP-based models often outperform traditional methods in algorithmic trading accuracy, reinforcing that machine intelligence can detect subtle signals better than rigid algorithms.
- Reinforcement Learning for Adaptive Strategies: One of the most exciting developments is using reinforcement learning (RL), a type of AI where an agent learns by interacting with an environment and getting feedback (rewards or penalties). In trading, the “environment” is the market and the “reward” could be profit. RL-based trading bots learn optimal behaviours through simulation and live trading, continually improving their strategy. This is a game-changer for market making and execution algorithms. An RL agent can learn how to adjust an order book’s shape to minimise price impact for large trades, or when to pull quotes to avoid being run over by sudden volatility. In one study, an RL-driven crypto trading strategy had fewer drawdowns and a higher win–loss ratio than a traditional rule-based approach, indicating it managed risk better and picked its trades more intelligently. Unlike static algorithms, an RL trader can decide not to trade during unrewarding market conditions, or aggressively provide liquidity when it’s most profitable, all based on its learned experience of the market’s behaviour.
- LLMs and Sentiment Analysis: Not all market-moving information is in price charts, especially in crypto, where tweets from a billionaire or a sudden regulatory news flash can send coins flying. AI now also means Large Language Models (LLMs) like GPT-4 being deployed to parse through news articles, social media and forums at scale. These AI models use natural language processing to gauge market sentiment and even predict how specific events might affect prices. For instance, an AI model could read thousands of Twitter posts about an upcoming Ethereum upgrade and determine whether traders are bullish or fearful, informing a strategy to go long or short. LLM-based systems can also summarise breaking news in milliseconds and feed the insights into trading algorithms, essentially bringing a fundamental analysis angle into high-speed trading. This kind of AI augments pure number-crunching with an understanding of the market’s mood, which is huge in a sentiment-driven arena like crypto.
- Optimising Trade Execution: AI isn’t just about deciding what to trade, but also how to trade. Execution algorithms, responsible for slicing up large orders and navigating multiple exchanges, are getting smarter with AI. Machine learning can learn the microstructure of each exchange (how orders typically fill, where hidden liquidity lies) and optimise order placement to reduce slippage. AI-driven execution bots might predict short-term price impact: for example, knowing that dumping a large order all at once will move the market 2%, they instead work the order in smaller pieces or route portions to different venues, adapting on the fly if volume spikes. High-frequency trading (HFT) in crypto is also benefiting: AI can react to fleeting arbitrage gaps or inefficiencies in a split second. An AI-powered market maker, for instance, can update its quotes across dozens of exchange order books in real time as soon as a big trade hits on one venue, preventing arbitrageurs from exploiting price lags. The outcome is tighter spreads and more efficient markets thanks to faster, smarter adjustments.
In short, AI is becoming the secret sauce for algorithmic crypto trading. It gives trading programs something resembling intuition: the ability to sense patterns or shifts in regime and adjust accordingly, rather than blindly following preset rules. And the impact is already being felt in measurable ways.
Proof in the Pudding: AI Outsmarting Traditional Quants
Does all this AI hype translate to better performance? The numbers say yes. AI-driven models have started to outperform more traditional quant strategies on multiple fronts, from profitability to risk management:
- Higher Returns and Better Precision: Hedge funds deploying AI-driven trading strategies have been beating their non-AI peers by an average of 12% in performance. The ability of AI to digest more data (including alternative data like social sentiment or on-chain metrics) and adapt to fast-changing conditions gives these funds a tangible edge. Even in more controlled strategies like arbitrage, machine learning has shown its worth, The International Monetary Fund noted that machine-learning-powered stat arb strategies delivered 5–7% higher returns in 2024 for leading funds compared to traditional tactics. In crypto trading, where volatility is higher and inefficiencies abound, similar or greater performance boosts are being observed by firms that lean into AI.
- Lightning-Fast Decisions: Speed matters in all financial markets, but in crypto’s HFT arena, it’s critical. AI models can recognise and react to a trading signal in milliseconds, far faster than any human. As mentioned earlier, top AI trading systems execute with 0.01s latency. This speed advantage can mean the difference between capturing an arbitrage opportunity and missing it. For example, if Bitcoin’s price jumps on Exchange A, an AI bot can instantly start buying on Exchange B (where the price hasn’t moved yet) and then sell on A, locking in profit before others even realise what happened. By contrast, a manual trader or slower system reacting in 0.3s or a full second is already too late; the gap has closed. This reduction in reaction time directly boosts profitability for market makers and arbitrageurs. It’s an AI arms race where the fastest and smartest algorithm wins.
- Rising Market Share of AI Trades: The majority of crypto trades are now executed by algorithms, and a growing chunk of those algorithms are powered by AI. As noted, over 70% of crypto trade volume is driven by automated trading bots, and many of the leading crypto liquidity providers have invested heavily in AI research. Exchanges themselves are partnering with AI-centric market makers to improve their order book quality. This increasing dominance of AI can be seen as self-reinforcing, AI traders provide tighter spreads and more volume, which attracts more traders, which then encourages more use of AI to stay competitive. It’s telling that even retail trading platforms now offer AI-driven trading bot features, touting higher win rates (some advanced bots claim over 80% trade success rate, though such figures should be taken with caution). The takeaway: AI is not niche in crypto; it’s quickly becoming standard equipment.
- Real Examples and Outliers: Several proprietary trading firms have hinted at the success of their AI models. While much is kept secret, there are anecdotes like a Sydney-based hedge fund replacing human analysts with AI and beating the stock market indices consistently. On the academic side, competitions and papers have demonstrated AI’s edge. For example, reinforcement learning bots in simulated crypto markets often achieve higher Sharpe ratios (risk-adjusted returns) than traditional strategies based on technical analysis. One prominent crypto fund wrote that their AI models helped reduce trading slippage by 10–20% and drawdowns by a similar margin compared to legacy algorithms (data that aligns with research observations of RL agents suffering fewer large losses). All this paints a clear picture: when properly trained and deployed, AI-driven trading systems are outmanoeuvring the old-school quants.
Of course, it’s not a total wipe-out, human quants and simpler algos still thrive in many niches. But the trend is that if you’re not augmenting your trading with AI, you’re likely giving up an edge to someone (or something) that is.
Benefits and Challenges of AI-Powered Trading
No disruptive tech comes without its pitfalls. AI in trading brings huge benefits, but also new challenges that both traders and regulators are grappling with. Let’s break down the key pros and cons:
Benefits
- Speed & Efficiency: As highlighted, AI can analyse data and execute orders in fractions of a second, an unparalleled speed advantage. It also never gets tired or emotional, an AI will diligently watch hundreds of markets at once and never miss a beat or make a typo. This efficiency means more opportunities captured and fewer mistakes. In volatile crypto markets, that can translate to significant profit gains simply by being faster and more precise.
- Adaptability & Learning: Traditional algos can falter when market regimes change (say, a shift from bull to bear market) because their rules were tuned for past conditions. AI models, by contrast, can adapt on the fly. They learn from new data continuously, adjusting their parameters or strategy when they detect that the market’s behaviour has shifted. This makes them more resilient in the face of crypto’s ever-evolving trends. An AI market maker can dynamically widen spreads during chaos and tighten them when markets calm, optimising its strategy in real time. Such adaptability was rarely possible at this scale before.
- Multi-Source Intelligence: AI can fuse disparate data sources for a more holistic market view. For example, it might combine price trends with sentiment from Twitter and even blockchain transaction data for an all-angle perspective. Humans struggle to monitor so many inputs simultaneously, but AI thrives on data. This multi-source analysis can reveal hidden patterns (like an uptick in on-chain activity foreshadowing a price rally) that a siloed approach would miss. It’s like having a hundred eyes on every aspect of the market at once.
Challenges
- Black Box Decisions: Many AI models, especially deep neural networks, act as “black boxes” and churn input into output in ways even their creators can’t fully explain. In trading, this lack of explainability can be problematic. If an AI starts making unusual trades, it’s hard to tell if it has found a genuine new pattern or if it’s making a mistake. This opacity not only worries the traders overseeing the bots but also regulators who are concerned about AI-driven market distortions. There’s active research into Explainable AI (XAI) to make these models more transparent, but it’s early days.
- Overfitting & False Signals: AI models are prone to overfitting; essentially, learning patterns that worked in historical data but won’t hold in the future. An AI might become over-optimised to the quirks of last year’s market data. When the market regime changes (as it always does in crypto), such a model can stumble badly. Overfitting is a well-known pitfall where a model fails to generalise and performs poorly on new data. In practice, this could mean an AI trader that was racking up profits suddenly starts haemorrhaging money when conditions change. Robust validation and continuous retraining are needed to avoid this, but the risk never zeroes out.
- Data Quality & Noise: Crypto markets produce a firehose of data, but not all of it is useful or even real. AI models can be led astray by bad data, whether it’s faulty price feeds, spoofed orders in the order book, or misleading social media rumours. Training on data that includes manipulation or random noise might cause the AI to “learn” patterns that are just noise. Separating signal from noise is tough, even for intelligent models. If an AI isn’t carefully monitored, it might, for example, overweight some bogus altcoin tweet as a trading signal. Human oversight and robust data filters remain crucial.
- Arms Race & Competition: As AI becomes the norm, having an AI might shift from a competitive advantage to a basic requirement. The more firms deploy similar AI strategies, the more those strategies compete and cannibalise each other’s profits. We could reach a point where many AIs converge on the same trades, reducing their effectiveness. Essentially, when everyone has a bot with brains, the edge becomes harder to find, leading to diminishing returns unless an innovation comes along. This arms race dynamic means AI gains could be temporary as the playing field evens out.
In summary, AI brings power and speed, but also complexity and new risk considerations. Savvy trading teams are managing these challenges with techniques like rigorous backtesting, risk limits for AI-driven trades, and “human-in-the-loop” oversight where algorithms must justify large moves to a human risk manager. The benefits, however, are enticing enough that the crypto trading world is plunging headlong into the AI era.
The Next 2–5 Years: AI’s Impact on Crypto Markets
So what’s on the horizon as AI continues to evolve in crypto trading? Expect the next 2–5 years to bring even deeper changes to market structure:
- Smarter and More Liquid Markets: As AI-driven market makers proliferate, even smaller crypto assets could see enhanced liquidity. Tiny altcoins that today have wild spreads and thin order books might, in a few years, be largely maintained by AI liquidity providers that can efficiently manage thousands of micro-market pairs. This could make the crypto market landscape more uniform and stable, with narrow spreads becoming standard even outside top coins. Paradoxically, markets may feel more “efficient” and less volatile in day-to-day trading (black swan events aside) because AIs are damping out inefficiencies and arbitrage gaps almost instantaneously.
- AI vs. AI Battles: With more AIs trading, we’ll inevitably see algorithms interacting, and even exploiting each other’s patterns. There may be instances of “adversarial trading,” where one AI tries to confuse or induce mistakes in another (imagine placing spoof orders to trick a competitor’s model). This could lead to a form of algorithmic warfare in the markets, with survival of the fittest algorithm. While humans used to play games of bluff, shortly AIs might be playing those games at a microsecond scale. The market could develop new forms of complexity as strategies evolve that no human would have designed explicitly, emerging from AI competition.
- Regulatory and Structural Changes: Regulators are already discussing oversight on AI in trading. We might see guidelines on testing AI algorithms before they go live to prevent erratic behaviour that could destabilise markets. Exchanges could implement circuit breakers specifically triggered by AI activity (for example, if an unusual pattern of orders suggests an algorithm gone rogue). On the flip side, AI might help regulators too, with surveillance AIs scanning for manipulation and abuses. The structure of crypto markets might shift towards more transparency, partly because AI thrives on data; projects might be pressured to release more on-chain info or disclosures since traders’ AIs will demand every edge in data they can get.
- New Financial Instruments and Strategies: AI might give rise to entirely new trading products. For example, derivatives that pay out based on an AI model’s performance or indexes tracking “AI sentiment”. We could see structured products that let investors essentially put money into an AI-managed crypto strategy easily (some early versions of this exist via copy-trading bots, but it could become more sophisticated and mainstream). In the high-frequency realm, strategies like latency arbitrage could become so optimized by AI that traditional HFT edges fade, leading traders to focus more on unique data sources (like satellite data for Bitcoin mining activity, or IoT sensors tracking global demand, any exotic data feed that an AI can ingest to predict markets).
Ultimately, the crypto markets in 2–5 years may look quite different. The game of trading could increasingly become AI vs AI, with humans overseeing the battleground from above. This doesn’t mean humans have no role: creativity, strategy design and risk management oversight are more important than ever, but the day-to-day execution and short-term decision-making will be dominated by algorithms that learn and adapt on their own.
For crypto investors and users, an AI-driven market could mean better liquidity, tighter spreads and perhaps a more stable trading experience most of the time. However, it could also mean that when things do go wrong, they go wrong in new and unpredictable ways (think flash crashes or bizarre feedback loops caused by duelling algos).
One thing’s for sure: AI is now part of the DNA of crypto trading. From deep learning models sniffing out price patterns to LLMs digesting the news, the intelligence of the machines is seeping into every corner of the market. The firms that harness it boldly (while respecting the risks) are likely to be the ones leading the pack in crypto’s next chapter. The crypto market has always been an innovation playground, and AI is its latest and perhaps most transformative toy – one that’s reshaping how markets are made and how trades are won.
References (Further Reading):
- Openware – The Role of AI in Crypto Market Making (May 2024) openware.comopenware.com
- Washington Beer Blog – How Crypto Bots Can Boost Your Trading Success (Nov 2024) washingtonbeerblog.com
- Autowhale – How new machine learning algos disrupt algorithmic trading in crypto (June 2024) autowhale.ioautowhale.io
- Clarigro – AI Impact on Hedge Fund Performance… What to Expect in 2025 (Dec 2024) clarigro.comclarigro.com
- MDPI Journal (Yang et al.) – Reinforcement Learning Pair Trading: A Dynamic Scaling Approach (2023) mdpi.com
- Medium (Saghiri) – Leveraging LLMs for Crypto Trading Bots (2023) medium.com
- Ankur’s Newsletter – The Future of AI Trading: algorithms, sentiments, and data (2023) ankursnewsletter.com
Contact Us
We are always open to discussing new ideas. Do reach out if you are an exchange or a project looking for liquidity; an algorithmic trader or a software developer looking to improve the markets with us or just have a great idea you can’t wait to share with us!